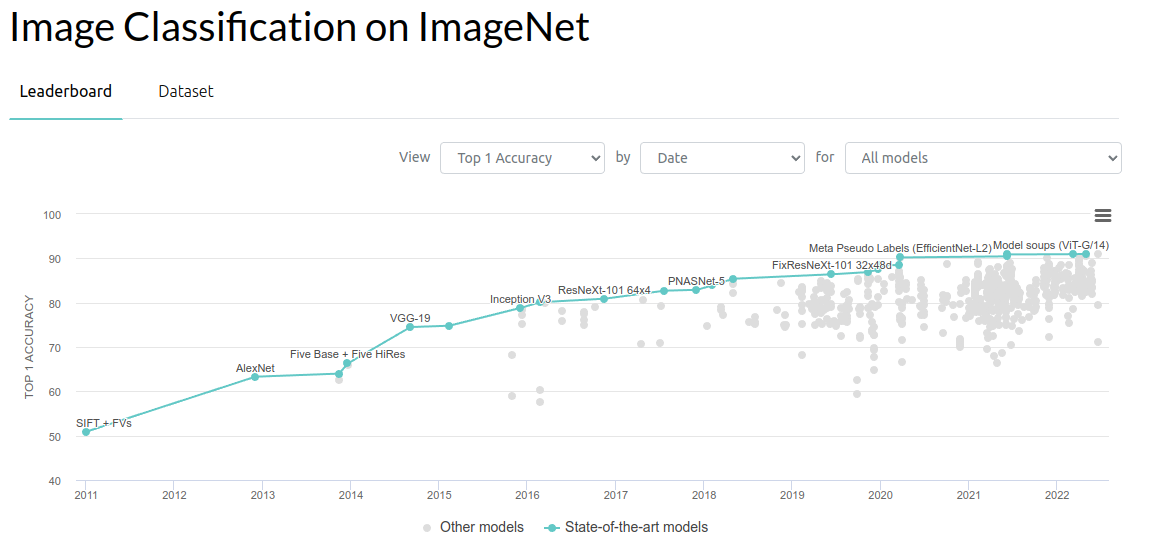
일반적으로 image classification 분야에서 새로운 model을 설계하는 것은 매우 어려운일이다.
그 이유는,
1) 단순하게 layer를 추가 구성해서 붙이는 과정으로는 model을 효율적으로 업그레이드 하는 것은 불가능함
2) 일반적으로 이미지 입력 크기의 경우 224-by-224 사이즈를 쓰게되는데 충분한 하드웨어 리소스 없이는 batch size를 백단위로 구성하는 것도 힘들며, batch size를 줄여서 학습을 하게 되면 모델 하나 학습하는데 몇일씩 걸리기 때문에 연구 개발 과정이 너무 길어지게 됨
따라서, image classification 분야에서는 주로 이미 개발된 뛰어난 model들을 가져와서 사용하게 된다.
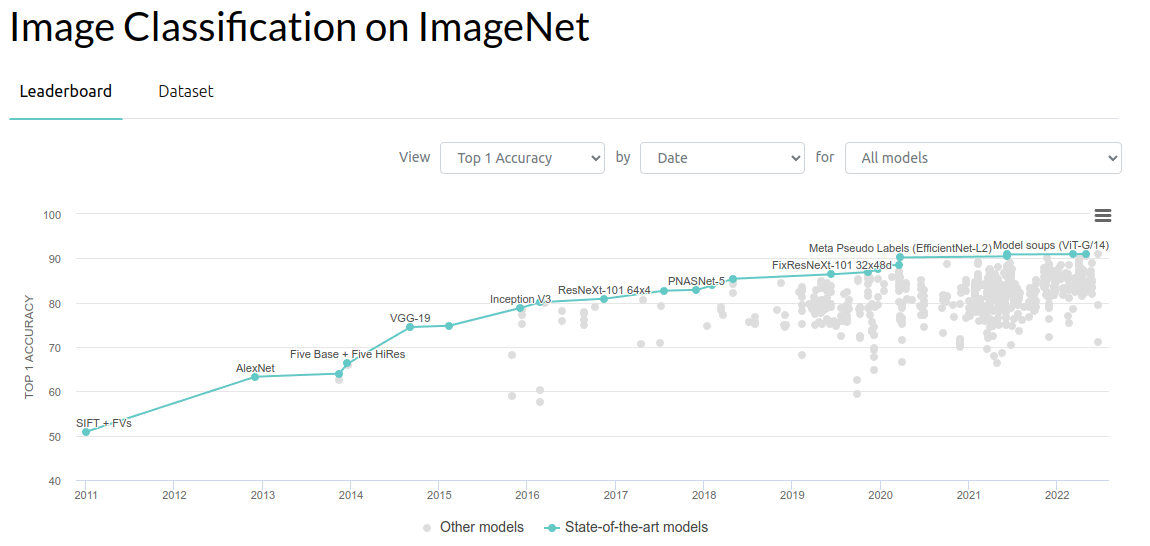
(출처: https://paperswithcode.com/sota/image-classification-on-imagenet)
이렇게 위와 같이 뛰어난 사전 학습된 model들을 pytorch에서 활용하기 위한 timm 모듈을 추천한다. (https://rwightman.github.io/pytorch-image-models/)
활용방법은 간단하다.
1) 설치
pip install timm
2) 활용 (ex: convnext_tiny)
import timm
net = timm.models.convnext_tiny(pretrained=True)
2-1) 클래스로 활용
from torch import nn
import timm
class LandmarkModel(nn.Module):
def __init__(self, **kwargs):
super(LandmarkModel, self).__init__()
backbone = kwargs["backbone"] # 백본 모델명이 입력되는 코드
num_classes = kwargs["num_classes"] # 분류하려는 이미지의 클래스 수
self.model = timm.create_model(
model_name=backbone, pretrained=True, num_classes=num_classes
) # 백본 모델 생성
def forward(self, inp):
x = self.model.forward(inp)
return x
일부 모델들은 pretrained=True를 사용할때 오류가 나기때문에 모든 모델을 활용하는 것은 불가능하지만
torchivision에서 제공하는 pretrained model보다 (https://pytorch.org/vision/stable/models.html)
timm이 더 많은 사전학습된 모델들을 제공하기 때문에 timm 모듈 사용을 추천한다.
(출처:huggingface)
아래는 사전학습된 사용가능한 모델들을 요약해놓았다.
Self-trained Weights
The table below includes ImageNet-1k validation results of model weights that I’ve trained myself. It is not updated as frequently as the csv results outputs linked above.
Model | Acc@1(Err) | Acc@5 (Err) | Param # (M) | Interpolation | Image Size |
efficientnet_b3a | 82.242 (17.758) | 96.114 (3.886) | 12.23 | bicubic | 320 (1.0 crop) |
efficientnet_b3 | 82.076 (17.924) | 96.020 (3.980) | 12.23 | bicubic | 300 |
regnet_32 | 82.002 (17.998) | 95.906 (4.094) | 19.44 | bicubic | 224 |
skresnext50d_32x4d | 81.278 (18.722) | 95.366 (4.634) | 27.5 | bicubic | 288 (1.0 crop) |
seresnext50d_32x4d | 81.266 (18.734) | 95.620 (4.380) | 27.6 | bicubic | 224 |
efficientnet_b2a | 80.608 (19.392) | 95.310 (4.690) | 9.11 | bicubic | 288 (1.0 crop) |
resnet50d | 80.530 (19.470) | 95.160 (4.840) | 25.6 | bicubic | 224 |
mixnet_xl | 80.478 (19.522) | 94.932 (5.068) | 11.90 | bicubic | 224 |
efficientnet_b2 | 80.402 (19.598) | 95.076 (4.924) | 9.11 | bicubic | 260 |
seresnet50 | 80.274 (19.726) | 95.070 (4.930) | 28.1 | bicubic | 224 |
skresnext50d_32x4d | 80.156 (19.844) | 94.642 (5.358) | 27.5 | bicubic | 224 |
cspdarknet53 | 80.058 (19.942) | 95.084 (4.916) | 27.6 | bicubic | 256 |
cspresnext50 | 80.040 (19.960) | 94.944 (5.056) | 20.6 | bicubic | 224 |
resnext50_32x4d | 79.762 (20.238) | 94.600 (5.400) | 25 | bicubic | 224 |
resnext50d_32x4d | 79.674 (20.326) | 94.868 (5.132) | 25.1 | bicubic | 224 |
cspresnet50 | 79.574 (20.426) | 94.712 (5.288) | 21.6 | bicubic | 256 |
ese_vovnet39b | 79.320 (20.680) | 94.710 (5.290) | 24.6 | bicubic | 224 |
resnetblur50 | 79.290 (20.710) | 94.632 (5.368) | 25.6 | bicubic | 224 |
dpn68b | 79.216 (20.784) | 94.414 (5.586) | 12.6 | bicubic | 224 |
resnet50 | 79.038 (20.962) | 94.390 (5.610) | 25.6 | bicubic | 224 |
mixnet_l | 78.976 (21.024 | 94.184 (5.816) | 7.33 | bicubic | 224 |
efficientnet_b1 | 78.692 (21.308) | 94.086 (5.914) | 7.79 | bicubic | 240 |
efficientnet_es | 78.066 (21.934) | 93.926 (6.074) | 5.44 | bicubic | 224 |
seresnext26t_32x4d | 77.998 (22.002) | 93.708 (6.292) | 16.8 | bicubic | 224 |
seresnext26tn_32x4d | 77.986 (22.014) | 93.746 (6.254) | 16.8 | bicubic | 224 |
efficientnet_b0 | 77.698 (22.302) | 93.532 (6.468) | 5.29 | bicubic | 224 |
seresnext26d_32x4d | 77.602 (22.398) | 93.608 (6.392) | 16.8 | bicubic | 224 |
mobilenetv2_120d | 77.294 (22.706 | 93.502 (6.498) | 5.8 | bicubic | 224 |
mixnet_m | 77.256 (22.744) | 93.418 (6.582) | 5.01 | bicubic | 224 |
resnet34d | 77.116 (22.884) | 93.382 (6.618) | 21.8 | bicubic | 224 |
seresnext26_32x4d | 77.104 (22.896) | 93.316 (6.684) | 16.8 | bicubic | 224 |
skresnet34 | 76.912 (23.088) | 93.322 (6.678) | 22.2 | bicubic | 224 |
ese_vovnet19b_dw | 76.798 (23.202) | 93.268 (6.732) | 6.5 | bicubic | 224 |
resnet26d | 76.68 (23.32) | 93.166 (6.834) | 16 | bicubic | 224 |
densenetblur121d | 76.576 (23.424) | 93.190 (6.810) | 8.0 | bicubic | 224 |
mobilenetv2_140 | 76.524 (23.476) | 92.990 (7.010) | 6.1 | bicubic | 224 |
mixnet_s | 75.988 (24.012) | 92.794 (7.206) | 4.13 | bicubic | 224 |
mobilenetv3_large_100 | 75.766 (24.234) | 92.542 (7.458) | 5.5 | bicubic | 224 |
mobilenetv3_rw | 75.634 (24.366) | 92.708 (7.292) | 5.5 | bicubic | 224 |
mnasnet_a1 | 75.448 (24.552) | 92.604 (7.396) | 3.89 | bicubic | 224 |
resnet26 | 75.292 (24.708) | 92.57 (7.43) | 16 | bicubic | 224 |
fbnetc_100 | 75.124 (24.876) | 92.386 (7.614) | 5.6 | bilinear | 224 |
resnet34 | 75.110 (24.890) | 92.284 (7.716) | 22 | bilinear | 224 |
mobilenetv2_110d | 75.052 (24.948) | 92.180 (7.820) | 4.5 | bicubic | 224 |
seresnet34 | 74.808 (25.192) | 92.124 (7.876) | 22 | bilinear | 224 |
mnasnet_b1 | 74.658 (25.342) | 92.114 (7.886) | 4.38 | bicubic | 224 |
spnasnet_100 | 74.084 (25.916) | 91.818 (8.182) | 4.42 | bilinear | 224 |
skresnet18 | 73.038 (26.962) | 91.168 (8.832) | 11.9 | bicubic | 224 |
mobilenetv2_100 | 72.978 (27.022) | 91.016 (8.984) | 3.5 | bicubic | 224 |
resnet18d | 72.260 (27.740) | 90.696 (9.304) | 11.7 | bicubic | 224 |
seresnet18 | 71.742 (28.258) | 90.334 (9.666) | 11.8 | bicubic | 224 |
'공부정리 > Computer Vision' 카테고리의 다른 글
[GAN] GAN 모델 안정화를 위한 기법 - LSGAN (0) | 2024.01.08 |
---|---|
[GAN] GAN이란? (0) | 2024.01.08 |
[GAN] VAE(Variational Auto-Encoder) (0) | 2024.01.05 |
[GAN] 확률 밀도의 추정과 샘플링 (1) | 2024.01.05 |
[Vision] albumentations라이브러리와 CutMix를 통한 이미지 증강 (0) | 2023.02.15 |